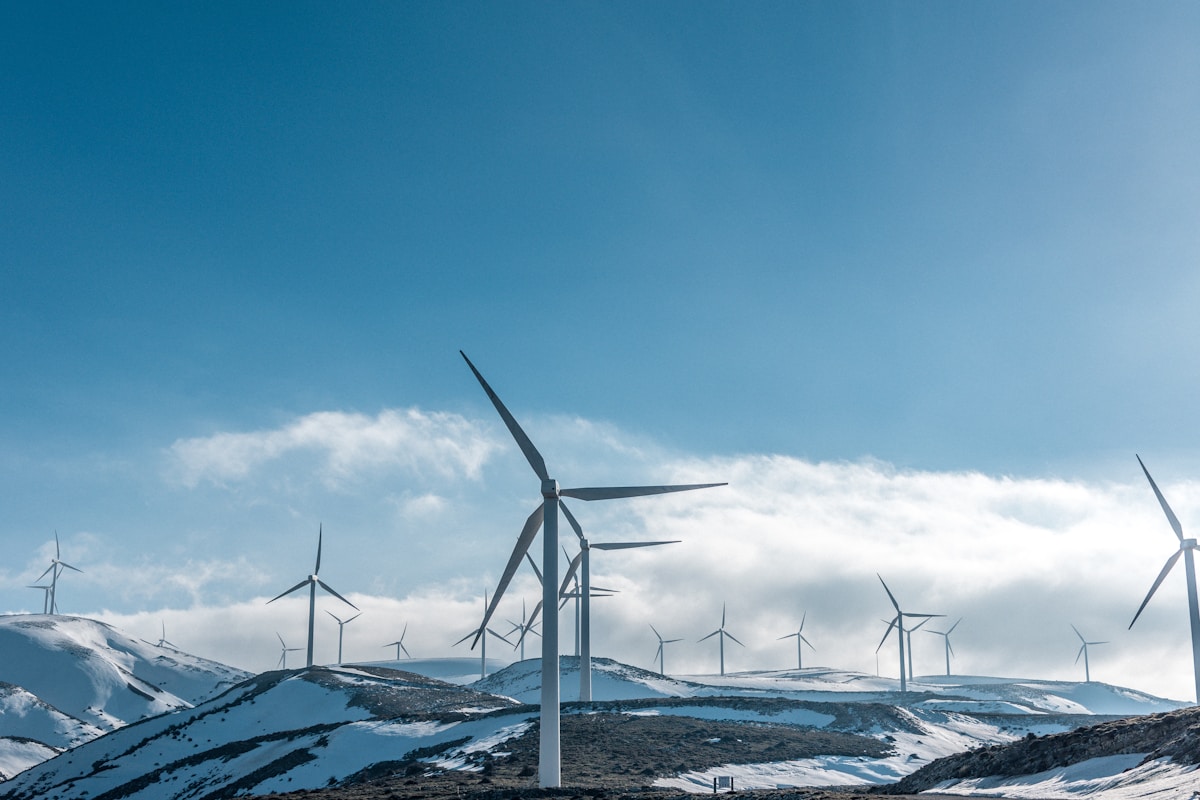
As the world becomes more data-driven and artificial intelligence (AI) continues to shape industries, understanding how technologies like cloud computing and edge AI differ is crucial. These two approaches to data processing and decision-making are transforming how businesses optimize performance, enhance real-time capabilities, and improve scalability. Let’s explore the key distinctions between cloud computing and edge AI, and why both are vital in today’s technology landscape.
Understanding Cloud and Edge AI
Cloud computing and Edge AI are reshaping how we process data and make decisions. Both technologies play vital roles in modern applications, offering unique benefits.
Cloud computing is all about handling and storing data over the internet. It provides massive computational power and storage, making it ideal for complex data processing tasks. This technology enables businesses to scale operations efficiently without hefty infrastructure costs.
Edge AI, on the other hand, processes data locally on devices like smartphones or IoT gadgets. This approach reduces latency and enhances real-time decision-making by minimizing the need to transfer data back and forth to the cloud. It's perfect for applications where speed and immediate responses are crucial.
Understanding these technologies is key to optimizing performance and efficiency. Here's why:
Scalability: Cloud computing supports extensive data processing and storage needs.
Speed: Edge AI offers faster data processing by handling information locally.
Cost-Effectiveness: Cloud solutions reduce the need for physical infrastructure.
Real-Time Decision Making: Edge AI excels in applications requiring immediate feedback.
Grasping the distinctions between cloud computing and Edge AI helps in selecting the right technology for specific needs, ensuring optimal performance and resource utilization.
Evolution of Cloud Computing
Cloud computing has transformed the way businesses manage data. It centralizes data processing and storage, providing scalable solutions that adapt to various needs. This technology allows companies to handle large volumes of information without the need for extensive physical infrastructure.
The development of cloud computing has led to significant benefits:
Scalability: Easily adjust resources to meet demand.
Cost Efficiency: Reduce expenses on hardware and maintenance.
Data Accessibility: Access information from anywhere with an internet connection.
Despite its advantages, cloud computing faces challenges. Latency can be an issue when data needs to travel back and forth between the user and the cloud. Bandwidth limitations may also impact performance, especially for applications requiring rapid responses.
These challenges have prompted the exploration of edge computing, which processes data closer to the source. By addressing latency and bandwidth issues, edge computing complements cloud technology and enhances real-time decision-making.
Understanding these developments helps in selecting the right approach for specific business needs. Both cloud and edge computing play crucial roles, offering distinct advantages depending on the application requirements.
What Is Edge Computing
Edge computing places computing resources closer to where data is produced. It enhances data processing by reducing latency and improving real-time capabilities. This approach processes information near its source, like on devices such as smartphones or IoT gadgets.
Edge computing addresses several cloud computing limitations:
Reduced Latency: Processes data locally, minimizing delays.
Enhanced Real-Time Processing: Ideal for time-sensitive applications needing immediate data handling.
Network Efficiency: Lowers network congestion by handling data at the edge.
By bringing processing closer to data sources, edge computing becomes essential for applications requiring instant responses. It effectively manages the challenges of network congestion and latency, making it a crucial technology for modern needs.
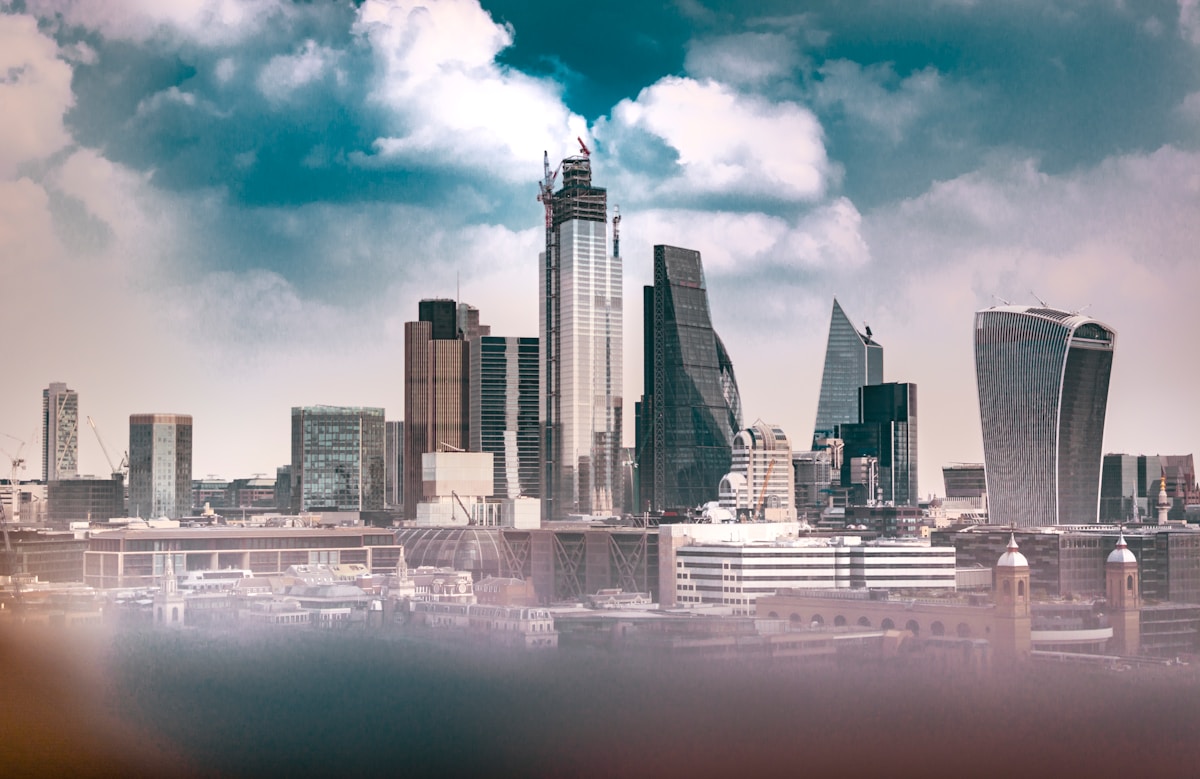
Cloud vs Edge AI
Cloud AI and edge AI serve distinct purposes in data processing. Each offers unique benefits suited to different application needs.
Cloud AI uses large-scale computations with extensive resources. It’s perfect for handling complex tasks that require significant computational power. This approach leverages centralized servers to process data efficiently, making it ideal for applications that don’t require immediate results.
Edge AI operates locally, on devices like smartphones or IoT gadgets. It processes data on-site, providing real-time insights with minimal latency. This is crucial for applications needing quick decisions, such as autonomous vehicles or smart home devices.
Key Differences:
Resource Usage: Cloud AI utilizes vast computational resources for heavy tasks. Edge AI works within the constraints of local devices.
Latency: Cloud AI may experience delays due to data travel. Edge AI offers lower latency by processing data on the spot.
Data Handling: Cloud AI is suited for large data volumes. Edge AI handles smaller, immediate data sets efficiently.
Choosing between cloud and edge AI depends on the specific needs of your application. Both technologies are powerful, but their effectiveness varies based on resource requirements and response times. Understanding these differences helps optimize performance and efficiency.
Benefits of Edge AI
Edge AI offers significant advantages by processing data locally. This reduces latency, making real-time insights possible. It's crucial for applications where immediate responses are needed.
Key Benefits of Edge AI:
Reduced Latency: Processes data on-site, minimizing delays and enabling quick decision-making.
Enhanced Reliability: Operates independently of cloud connectivity, ensuring consistent performance even with network issues.
Improved Data Privacy: Keeps data local, reducing exposure and enhancing security by minimizing the risk of breaches.
Lower Costs: Cuts cloud communication expenses by handling data processing at the source, reducing the need to send large amounts of data to the cloud.
Edge AI's ability to provide real-time insights is invaluable. It supports applications like autonomous vehicles and smart home devices, where speed and accuracy are essential. This localized processing boosts efficiency and reliability, offering a robust solution for modern technological needs.
Challenges with Cloud Computing
Cloud computing has its perks, but it's not without challenges. Bandwidth is a big one. Transferring large amounts of data can be slow and costly. This can lead to delays, especially when speed is crucial.
Latency is another issue. When data travels long distances to a central server, it takes time. This delay can impact applications where real-time processing is essential.
Network disruptions are also a concern. If the connection drops, access to data and services can be lost. This can hinder productivity and lead to frustration.
Costs add up too. Storing and processing data in the cloud can become expensive, especially for businesses with large-scale needs.
These challenges highlight why edge computing is gaining traction. By processing data closer to where it's generated, edge computing reduces latency and bandwidth usage. It offers a more efficient and reliable solution for real-time applications.
Understanding these limitations helps in making informed decisions about using cloud and edge technologies effectively.
Real-World Applications of Edge AI
Edge AI is making waves in various industries by providing real-time data processing and immediate decision-making. This localized approach is enhancing efficiency and innovation across multiple sectors.
In security systems, edge AI processes data from cameras and sensors on-site to detect threats instantly. This quick response is crucial for maintaining safety and preventing incidents.
IoT devices benefit from edge AI by analyzing data directly on devices like smart thermostats or appliances. This allows for immediate adjustments and optimizations, improving user experience and energy efficiency.
In healthcare, diagnostics powered by edge AI enable real-time analysis of patient data. This means faster results and more accurate treatments, which can be life-saving in critical situations.
Autonomous vehicles use edge AI to process the vast amounts of data generated while driving. This ensures rapid decision-making for navigation and obstacle avoidance, enhancing safety and performance.
Industrial automation sees edge AI streamlining operations by predicting equipment failures and optimizing production processes. This increases productivity and reduces downtime.
Edge AI is transforming industries by enabling quicker responses and enhancing productivity through localized data processing. Its ability to process information directly at the source is driving new innovations and efficiencies.
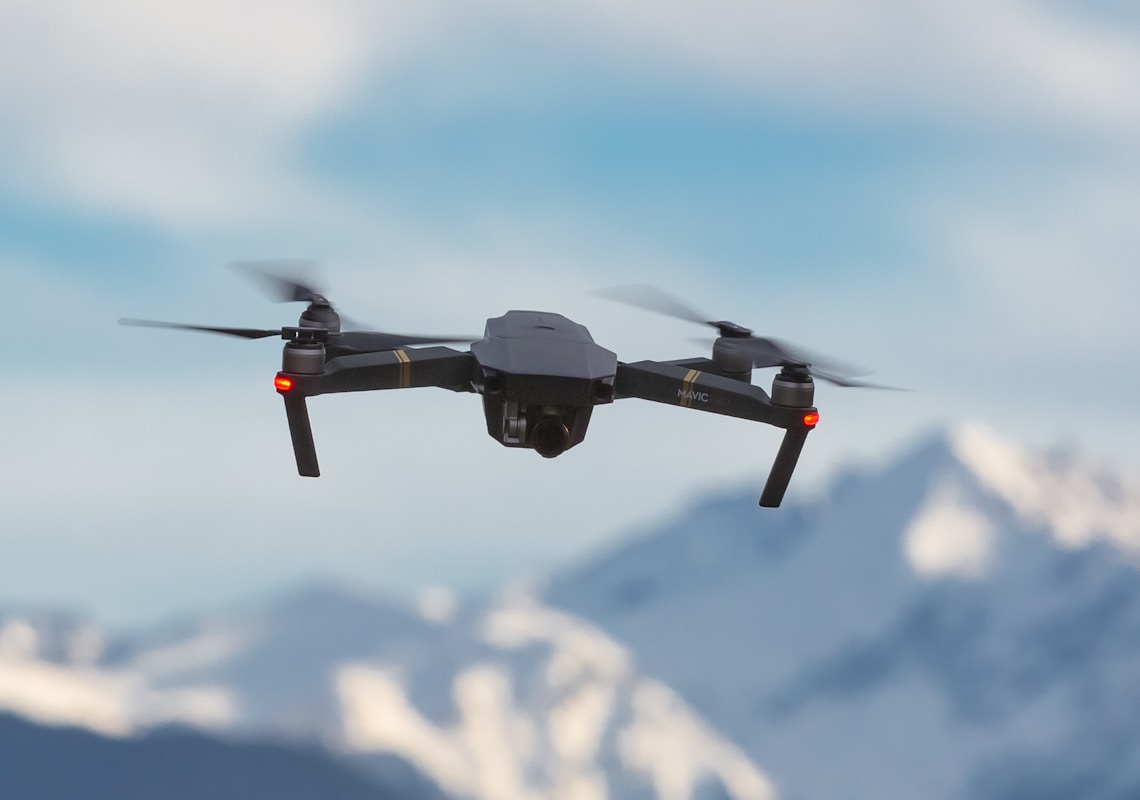
Integrating Cloud and Edge AI
Cloud and Edge AI work together to boost efficiency and performance. Cloud computing helps Edge AI by training models and gathering data, making AI more effective.
The cloud handles most data processing. It trains models with massive computing power, creating accurate and strong AI. These models then go to edge devices for real-time use.
Edge AI shines at quick insights. It processes data on-site, cutting down cloud communication. This works great for apps needing fast responses, like smart devices and self-driving systems.
Here's how to use both technologies for best results:
Better Performance: Use cloud for big calculations and edge for quick data processing.
Easy Growth: Cloud grows computing power, while edge adds more processing spots.
Save Money: Cut data transfer costs by processing locally with Edge AI.
Adapt Easily: Meet different needs by mixing central and local processing.
Using cloud and edge AI together helps businesses build flexible, robust solutions. This combo leads to efficient, scalable, and cost-effective AI operations.
Key Takeaways
Understanding cloud computing and edge AI is essential for optimizing technology use. Each has unique benefits that cater to different needs.
Cloud Computing: Offers vast computational power, ideal for processing large data sets and complex tasks. It helps businesses scale efficiently by centralizing data storage and processing.
Edge AI: Processes data locally on devices, reducing latency and improving real-time decision-making. It's perfect for applications requiring immediate responses, like smart devices and autonomous systems.
These technologies are crucial for enhancing performance and efficiency. Cloud computing provides the backbone for large-scale operations, while edge AI delivers speed and immediacy.
Consider how these insights fit with your needs. Whether managing data-intensive processes or requiring quick responses, understanding these technologies can help you make informed decisions.
For a broader understanding of how AI is transforming industries, check out our article on The Rise of AI at the Edge.
Comments